This talk gives an overview of three major use cases for multilingual RMarkdown: building self-documenting data pipelines, rapidly prototyping data science assets, and building ad hoc reports. Our focus is on why multilingual Rmd is valuable *in addition to* the reasons Rmdis already a valuable format (a good general case for Rmd exists here.) The case for multilingual Rmd focuses on flexibility, collaboration, time-to-value, and indecisiveness (in a good way!). Three examples demonstrate why multi-lingual Rmd should be a part of a data scientist’s toolkit.
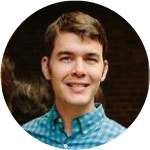
Aaron’s background is in building business processes and data systems for commodity companies. Most recently he used R to automate finance, risk management, and reporting activities for a coffee trading business.