What you’ll learn:
At RStudio, we believe successful data science teams require the right technology: open source, code-first data science frameworks based on R and Python, centralized on-prem or in the cloud for ease of collaboration, sharing and administration. However, technology on its own is not enough. It is just as critical to focus on the people in your team. To have a sustainable impact on decision making in your organization, you need to build a productive, effective, and collaborative data science team.
In this panel webinar, you will hear from leaders at Warner Music Group, T-Mobile, Saturn Cloud, The Looma Project, and Caliber Home Loans, about what it takes to build a successful data science team:
- Their experiences and perspective on building great data science teams of both R and Python users
- How their teams prioritize and deliver work in a way that adds value to their organizations, while also maintaining credibility among stakeholders even when the results aren’t necessarily good news
- Their advice for advancing your own data science career and collaborating with non-data-science stakeholders
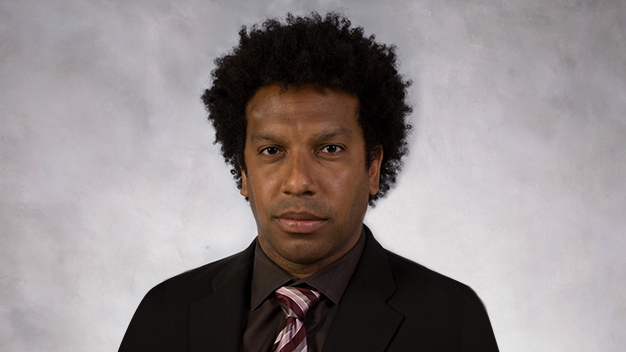
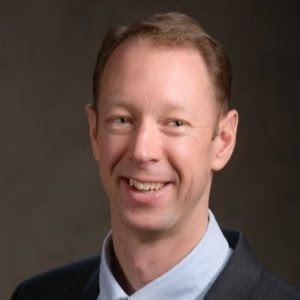
Greg is an innovative and dedicated analytics leader with 20 years of advanced data analysis experience, heading up a quickly expanding data science team of R and Python users at Caliber Home Loans. Greg has over 30 peer reviewed publications spanning the Economics, Statistics, and Medical domains.
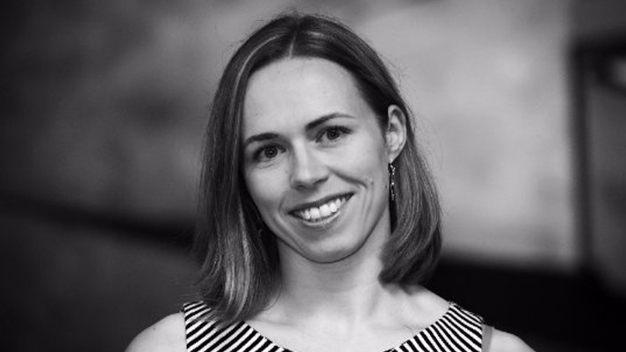
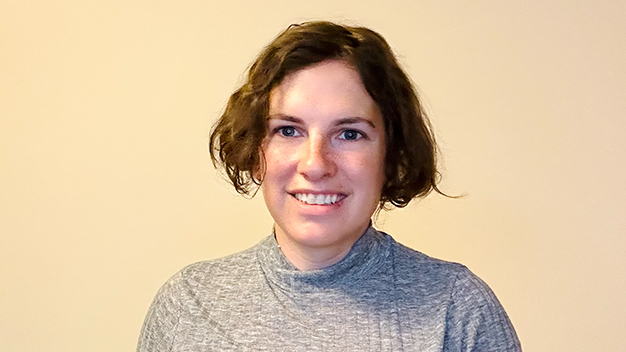
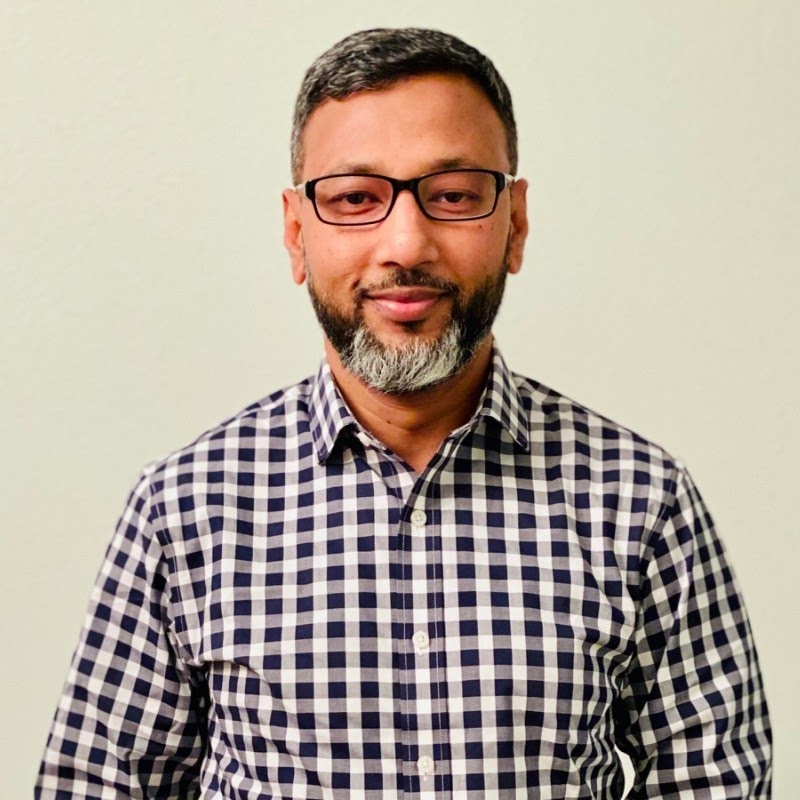
Nasir is a thought-leader who has built, trained, and retained exceptional data & analytics teams for increasingly better customer experience, with a recent focus on the uniting of two teams given the T-Mobile and Sprint merger. He is passionate about delivering self-service analytics solutions that empowers business users in making quality decisions.
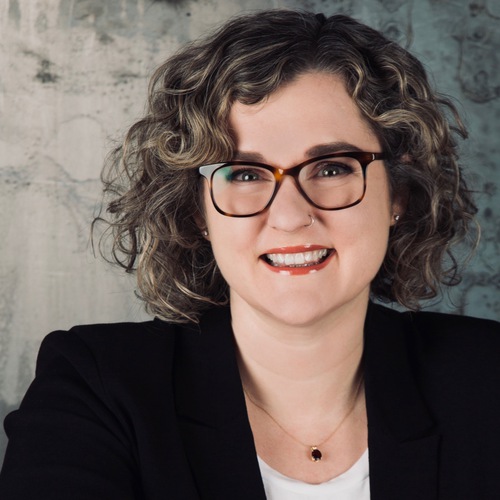
Julia Silge is a data scientist and software engineer at RStudio PBC where she works on open source tools for machine learning and MLOps. She holds a PhD in astrophysics and has worked as a data scientist in tech and the nonprofit sector, as well as a technical advisory committee member for the US Bureau of Labor Statistics. She is a coauthor of Tidy Text Mining with R, Supervised Machine Learning for Text Analysis in R, and Tidy Modeling with R. An international keynote speaker and a real-world practitioner focusing on data analysis and machine learning, Julia loves text analysis, making beautiful charts, and communicating about technical topics with diverse audiences.