R has an excellent framework for specifying models using formulas. While elegant and useful, it was designed in a time when models had small numbers of terms and complex preprocessing of data was not commonplace. As such, it has some limitations. In this talk, a new package called recipes is shown where the specification of model terms and preprocessing steps can be enumerated sequentially. The recipe can be estimated and applied to any dataset. Current options include simple transformations (log, Box-Cox, interactions, dummy variables, …), signal extraction (PCA, ICA, MDS), basis functions (splines, polynomials), imputation methods, and others.
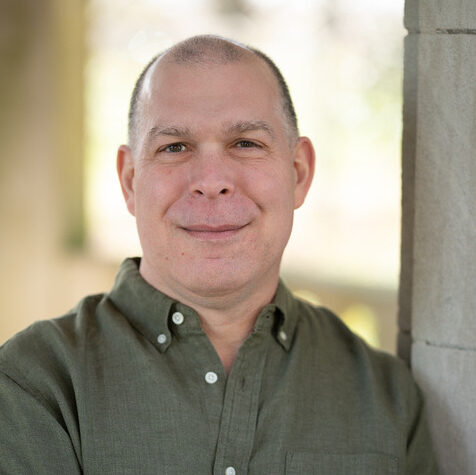
Max Kuhn is a software engineer at Posit (née RStudio). He is working on improving R's modeling capabilities and maintaining about 30 packages, including caret. He was a Senior Director of Nonclinical Statistics at Pfizer and had been applying models in the pharmaceutical and diagnostic industries for over 18 years. Max has a Ph.D. in Biostatistics. He, and Kjell Johnson, wrote the book Applied Predictive Modeling, which won the Ziegel award from the American Statistical Association. Their second book, Feature Engineering and Selection, was published in 2019, and his book Tidy Models with R, was published in 2022.