Measuring success of data science
Lindsey Clark
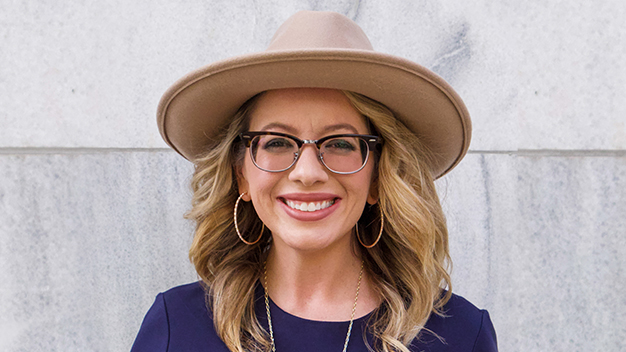
Episode notes
We were joined by Lindsey Clark, Director of Data Science at Healthcare Bluebook. Evolving and Leading a Data Science/AI First Organization.
One of the topics of discussion (33:46) was measuring the success of a data science team and calculating ROI.
How do you measure success for a data science team?
Lindsey shared a few tips from her experience at two different organizations:
One measure of success was the conversion rate of projects. How many projects come to us and what percent are actually converted to an actual deployment? Our target was 5% which may sound low, but the idea was that we want to fail fast. It can be like the drug discovery mentality – if things aren’t going to work, we want to know early on.
We also have targets around the number of specific models we wanted – what we called our data science portfolio library. They didn’t need to be really fancy machine learning, but a lot of them were business logic sort of ranking models.
Another measure was product value and financial targets around that. Could we pinpoint that our model went into the data product and it resulted in more clients contracting with us or greater revenue because we were able to upsell a data product due to another feature we added.
In my position now, we have recently been acquired so we are working on the success measures and what that looks like. We do utilize the Outcomes and Key Results (OKR) Strategy from the Measure What Matters book.
We plan OKRs quarterly and try to plan projects that are really no longer than three month increments in order to keep things going and satisfy the business.
Data Science ROI can of course be a difficult thing to measure. Data science can be a capital expense at times and sometimes you have to look at this team that’s going to do high risk projects as research. Things are going to fail and that’s okay. We’re willing to invest the money for our team and absorb that cost for what we potentially get out of the team in terms of deployed solutions, specific insights, and research that are valuable to the business.
Featured in this episode
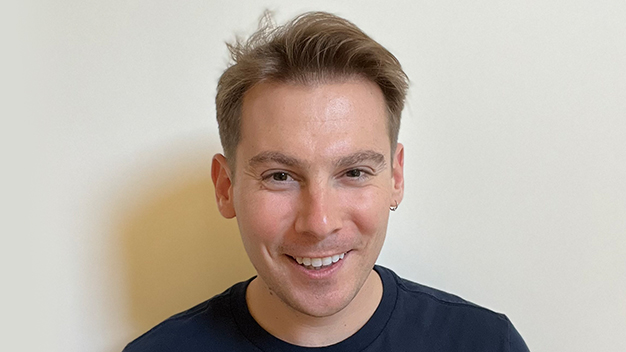
I lead demand generation and an incredible team at RStudio. We make the best open-source and professional software for data scientists. I am passionate about telling exceptional stories at scale, running effective digital programs, and delivering wonderful experiences for prospects and customers.
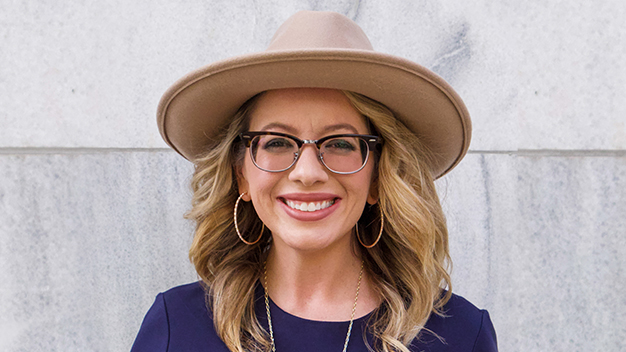
Experienced strategic leader and data professional with demonstrated success in data science strategy, machine learning (unsupervised and supervised techniques), regression and classification techniques, statistics, and end-to-end, deployed analytical solutions. Highly focused on value creation and user experience. Experience in client-facing situations. Focused on actionable and interpretable data solutions and building high performance teams. Deep experience in health outcomes, healthcare quality measurement, medical claims analytics, ROI demonstration.