Insurers commonly rely on standardized statistical methodologies to evaluate risk and determine insurance premiums. This assessment often entails the use of enterprise software, which can, however, constrain insurers to predefined methods and data types embedded within these solutions. The primary conventional data sources typically include customer portfolios and their claims history. Employing the classical frequency-severity method, insurers can estimate the anticipated number of claims and their associated costs, enabling them to set appropriate premium rates.
Smart Pricing with Posit Workbench for one of Germany’s largest insurance companies
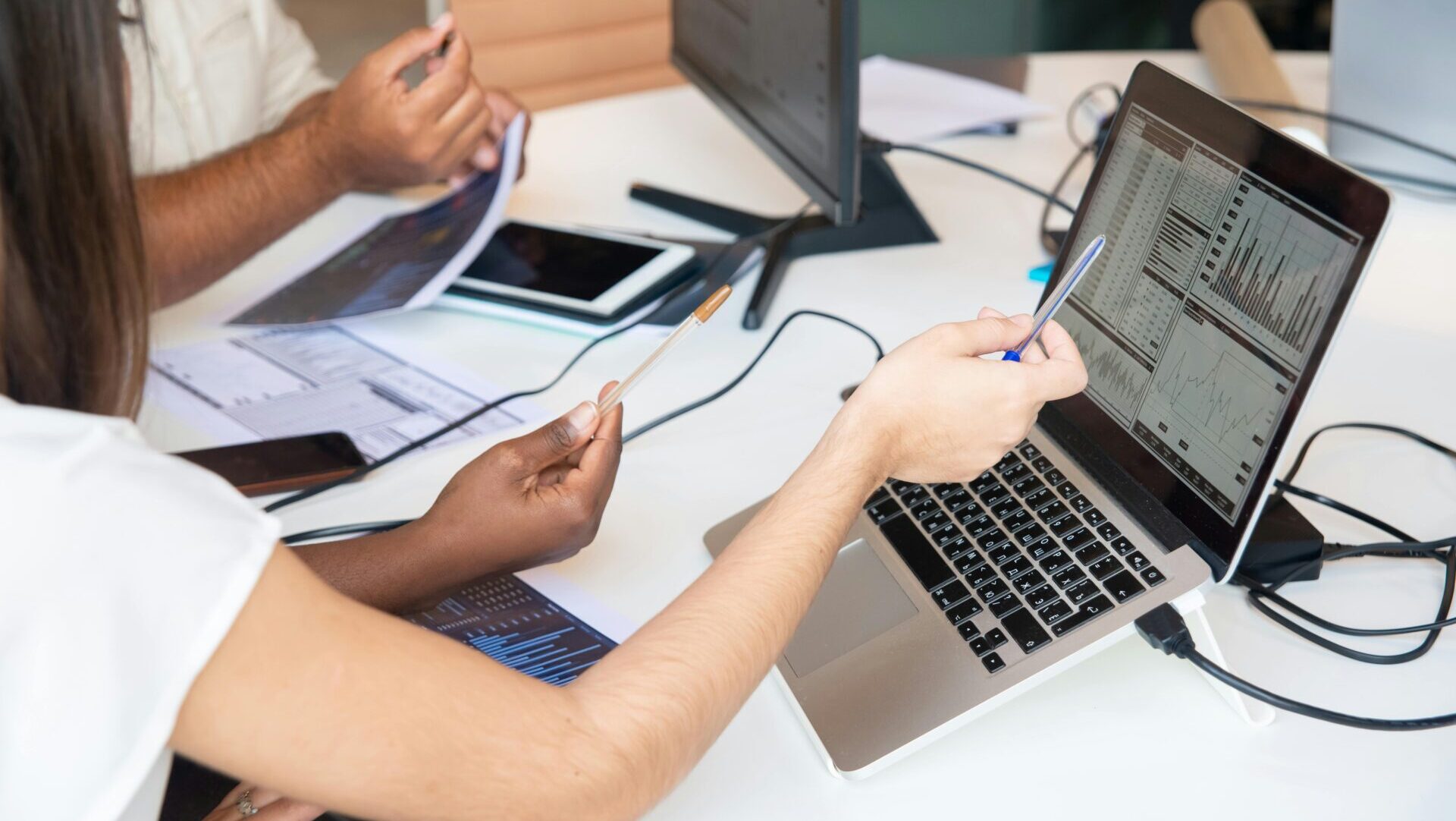
Introducing Smart Pricing
While it remains imperative to accurately evaluate risks and appropriately price premiums based on corresponding risk levels, a comprehensive pricing process should consider a myriad of factors, wherever feasible under legal regulations. These factors include:
Demand: Beyond analyzing past sales and customer acquisition, it’s essential to delve into segments interested in your products that haven’t yet converted into customers. This entails examining various touch points along the customer journey. It is possible that price is a determining factor preventing prospective customers from making a purchase.
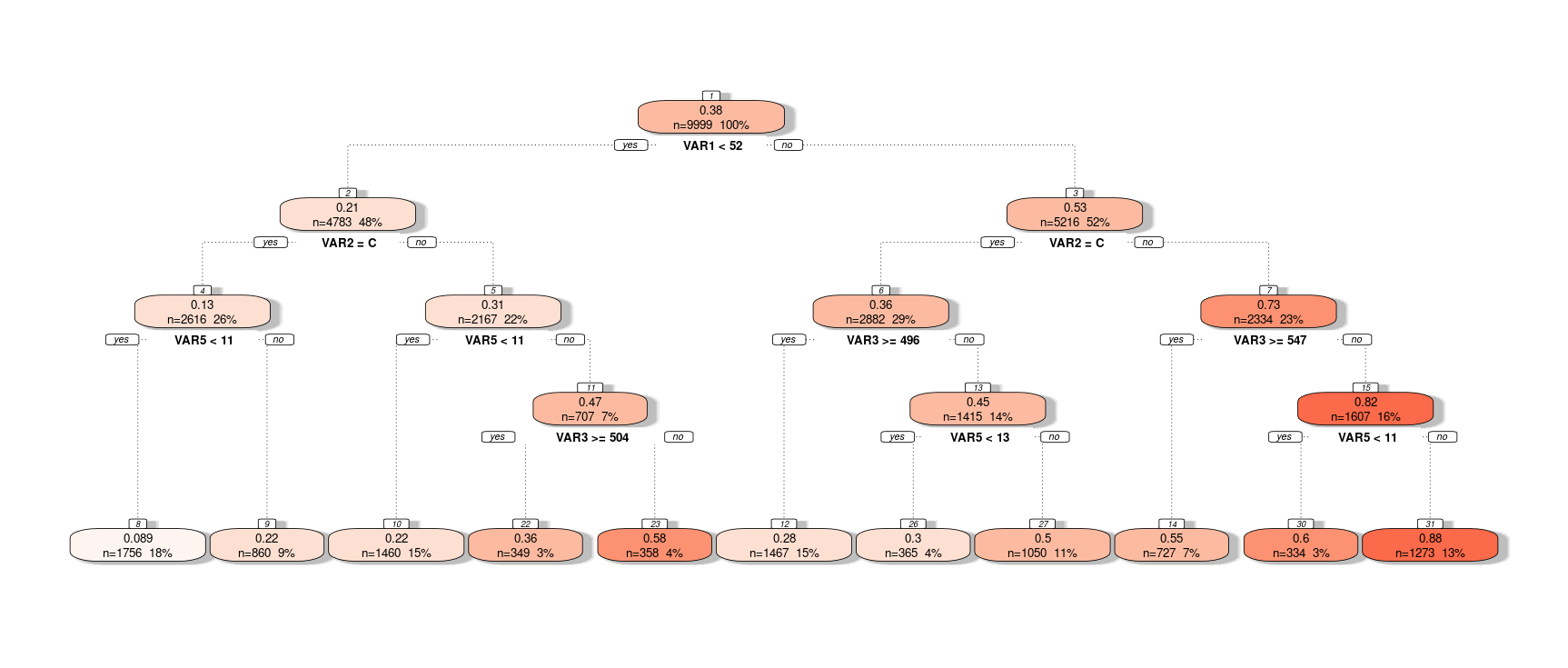
Contract Duration: Many contracts extend over several years, during which the risks and premiums may fluctuate significantly. For instance, in motor vehicle insurance, the likelihood of claims decreases notably after the first year, while premiums often rise with successive renewals. To determine the appropriate price, it is essential to forecast customer retention patterns and assess their likelihood of remaining within the portfolio throughout the full duration. This is essential for justifying the incurred costs of acquisition and anticipated claims.
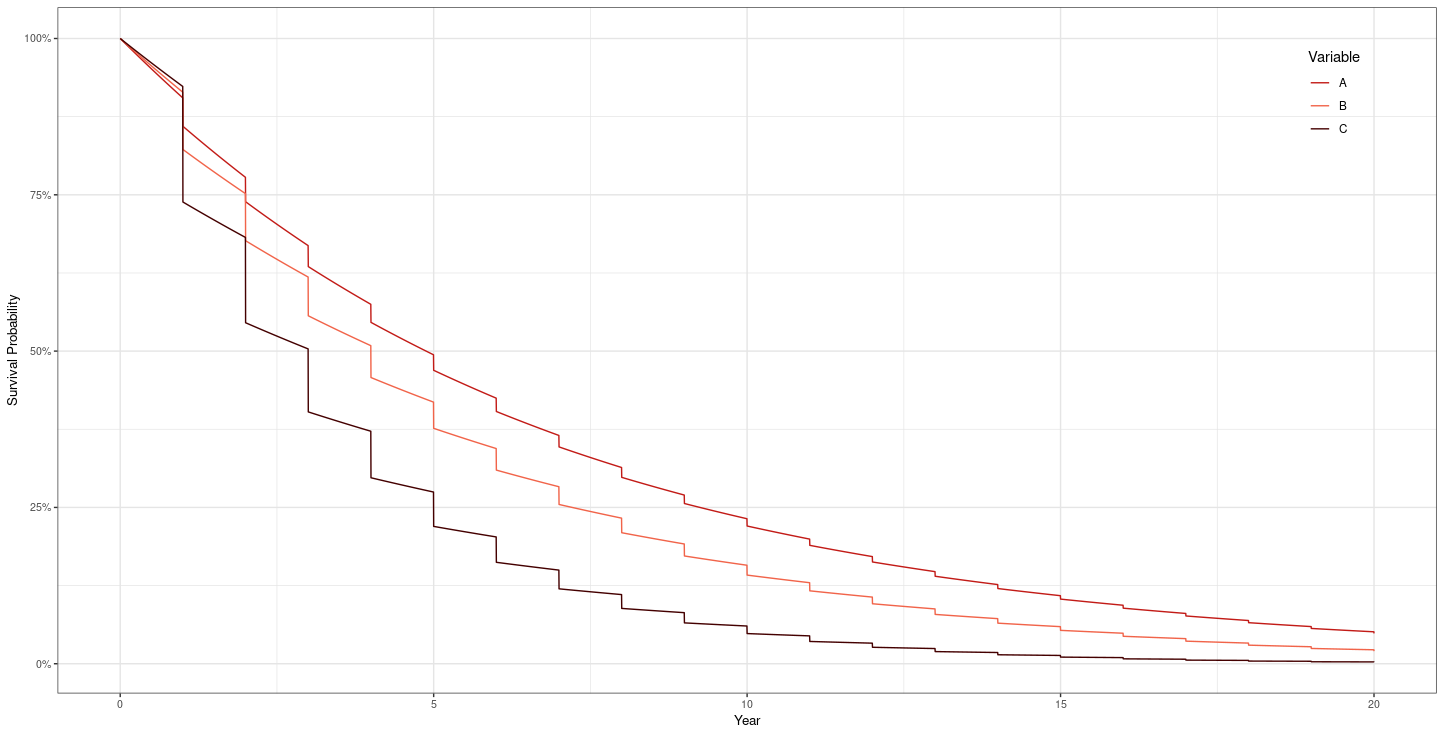
Price Sensitivity: The significance of price and services varies across products and customer segments. Understanding how price influences conversion probabilities in specific segments is key to selecting an appropriate pricing strategy. In segments where price is paramount, even minor discounts can drive substantial new business, potentially offsetting increases in less price-sensitive segments.
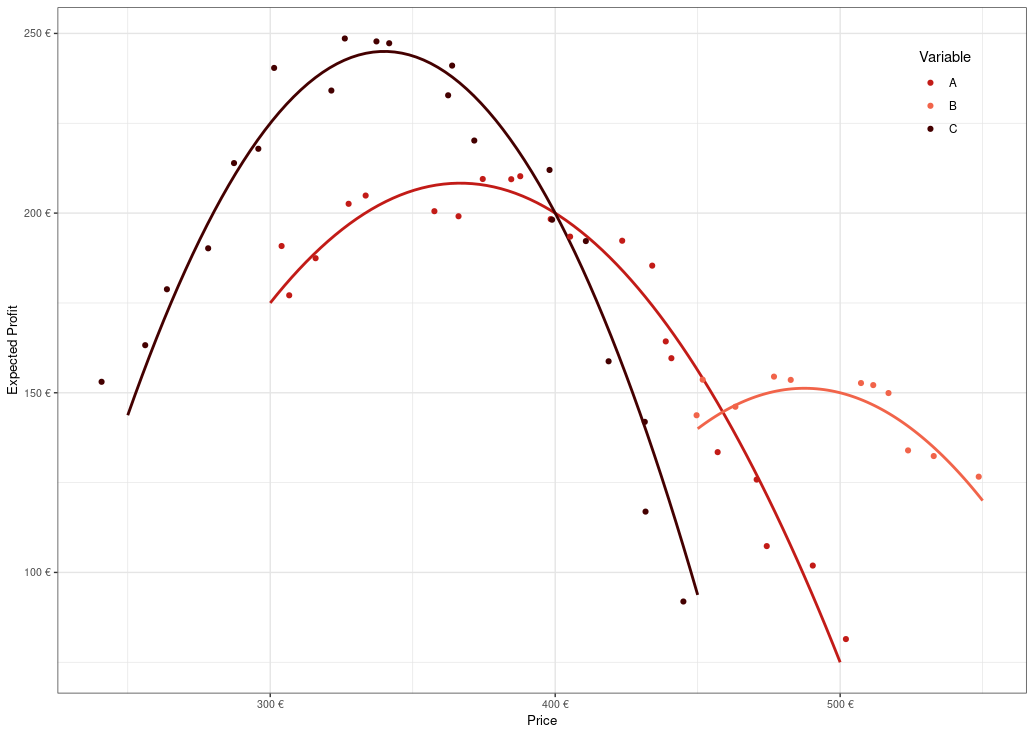
Analytics
By leveraging open-source data science and Posit Workbench, CosmosDirekt enhanced their pricing methodologies and delivered substantial value-added outcomes. Their approach encompassed several critical components:
- Establishing a robust data foundation, whether by sourcing existing data or designing experiments to generate new insights, to effectively address the challenges at hand.
- Employing a suite of modeling tools tailored to various data science problems, with a focus on classification, regression, and survival analysis techniques.
- Deploying the solutions into operation
- Implementing monitoring mechanisms to track model performance and insightful reporting of the results
Integration with their actuarial pricing suite required the conversion of models into PMML (Predictive Model Markup Language) format, utilizing specific R packages.
Posit Workbench allowed the team to focus on data science tasks, while reducing the administrative burden needed to manage their working environment. The ability to use multiple versions of R, coupled with renv, facilitated the development of robust solutions that could be easily shared across the team. Prior to this, updating packages posed a risk of breaking functionality and demanding considerable attention from the team.
The ability to open multiple sessions in Posit Workbench enhanced productivity, enabling their data scientists to work on several projects simultaneously. This capability was indispensable for addressing the diverse tasks inherent in the smart pricing project, encompassing the development of various models, data collection and exploration, as well as monitoring and reporting.