Exploring team structure (data scientists, data engineers)
Michael Chow
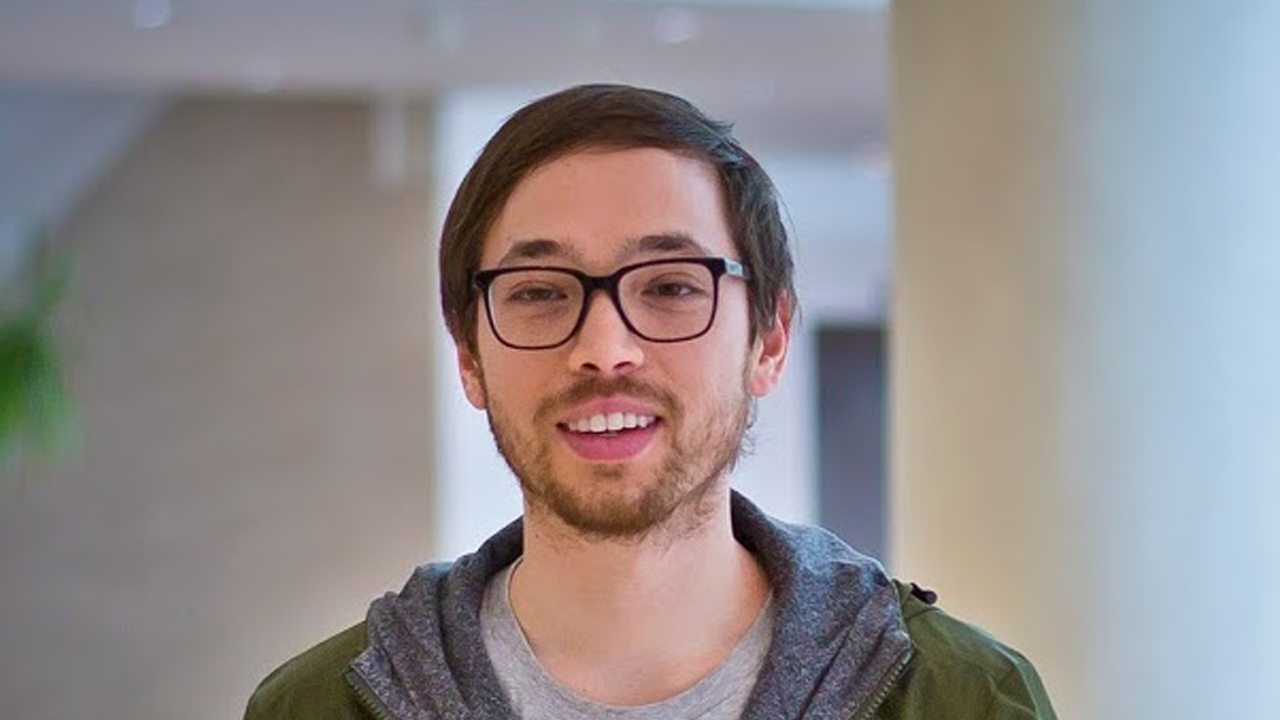
Episode notes
We were joined by Michael Chow, Data Scientist and Software Engineer at RStudio. Michael also previously led a team at the California Integrated Travel Project.
On this week’s hangout there were a lot of thoughts shared on structuring a data science team from both Michael and the broader group:
⬢ Jacqueline Nolis also shared thoughts on this on a data science hangout that there were virtues to different ones, but ended up sold on the decentralized model where data scientists are embedded in teams: https://youtu.be/CcPE29bYGVo?t=325
⬢ Michael agreed that data scientists and analysts should be sitting with the teams that they’re pushing out reports for. Otherwise, I would be trying to send people into those teams to figure out their priorities.
⬢ A data scientist should work with a Project Manager or whoever’s leading the team to push up metrics but also help change the roadmap.
⬢ It leaves a tricky question of where data engineers should be and how they should interact with the team. Today data engineers are often doing more tooling empowerment, so it can be okay to have them a bit more centralized and connect to the data scientists to enforce best practices or enable new pieces for them.
⬢ I think a nice model is for data scientists/analysts to live in the teams and data engineers to be like spokes of a wheel where then the data scientists connect with them and work closely to enforce better best practice and enable new important things.
⬢ Tatsu shared that in thinking of the structure, it’s also important to find your translators and to use the power of feedback. Reach out to those people to start to put that feedback into action.
⬢ George shared that insurance companies have come from a really traditional landscape where they have lots of actuaries working on lots of excel spreadsheets and there can be a lack of knowledge sharing and tool sharing. This is where the data science element comes in. To me, within the organization, you need to have this team which is a mini-spoke if you will, because they are central to the actuarial team. If they are too far removed and they’re back with the IT team, you end up with the old problems because they may not get the business concept communicated back. It’s all about getting enough skills, so they can get stuff done, especially proof of concepts. Maybe after that you can take a step back and then start to look at the centralized model again.
⬢ A central team can help converge to what they see as best practice, but if you’re pushing out something new, exploring a new line of work or area it can be important to set the data engineer there to actually do whatever they need to. Make sure that the converging doesn’t stifle creativity or prevent a team from doing the right thing.
⬢ Manny jumped in to share the perspective from data science being with IT as well, data science is a new field for their company (in real estate) and there’s an identity of where does data science fall. The IT team is fantastic and they’re very structured. Data science is so fluid and creative and non structured at the moment, so you kind of have to look at where it actually should fall.
💡Resources shared:
⬢ Tatsu shared in the chat, a few projects that Michael is working on: vetiver: https://vetiver.tidymodels.org/articles/vetiver.html, siuba: https://github.com/machow/siuba
⬢ Libby shared a helpful tip on creating a 2 minutes YouTube video with a cover letter, to get the attention of a hiring manager
⬢ Javier shared an example Shiny app used in an interview: https://javierorraca.shinyapps.io/Bloomreach_Shiny_App/
⬢ Michael mentioned David Robinson’s screencasts: https://www.youtube.com/channel/UCeiiqmVK07qhY-wvg3IZiZQ
⬢ Michael mentioned an article on “What data scientists really do according to 35 data scientists”: https://hbr.org/2018/08/what-data-scientists-really-do-according-to-35-data-scientists
⬢ Rachael shared a blog post link where Jacqueline Nolis talked about team structure as well: https://www.rstudio.com/blog/building-effective-data-science-team-answering-your-questions/#Structure
Featured in this episode
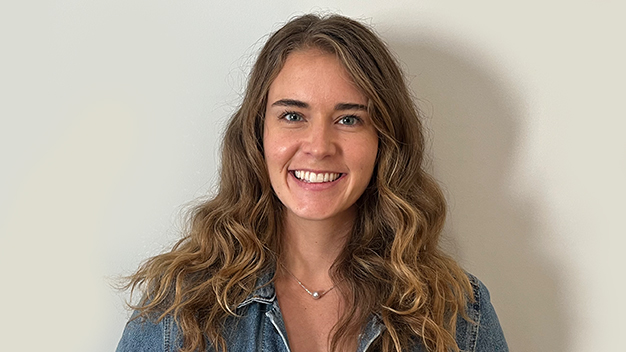