Partnership-model when forecasting
Dara Straussman
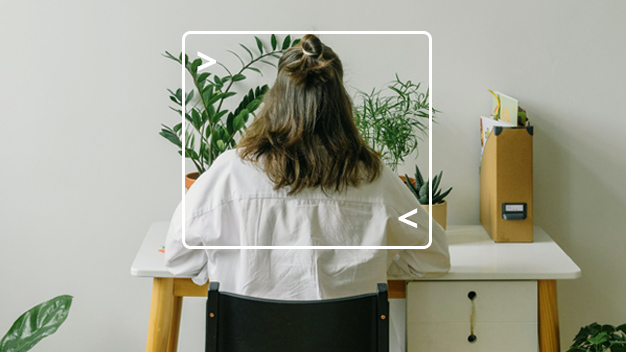
Episode notes
We were joined by Data Straussman, Data Science Manager at Stripe. Dara’s team focuses on forecasting, machine learning, and analytics in the Finance and Operations spaces.
We were able to get a glimpse into their financial modeling methodology at Stripe after a question from Alan at 12:59.
Question: How do you get buy-in from business stakeholders who historically would have been setting targets (like salespeople and their leaders) that the forecasting you’re doing seems like a realistic enough picture of the world?
Answer: It’s an ongoing evolution for how we do this interaction because it’s very important to get the input from stakeholders.
There might be a big sales deal coming down the pipeline that no statistical model will know about. There might be some product launch plans that we’re just not going to be able to learn from history. So we find that interaction very important.
When we started doing the – we call it the statistical forecasting and the adjustments — it was all done in spreadsheets by finance folks. We did really have to prove our value and show how a statistical model will actually help.
So our current system:
1. We have the statistical model that’s all done by data science.
2. We do backtesting and reports to be able to build confidence in each level of the hierarchy so that people can understand where do they expect accuracy, where do they expect less accuracy.
3. We have a whole process with the finance and strategy team, where they make adjustments to the forecast and are pretty clear about where they are doing adjustments and how it’s affecting the total.
4. Then we track both – the statistical versus the adjusted forecast. What we’ve learned over time is that making adjustments in certain ways or certain places is very important and very effective. We sort of encouraged the finance and strategy team to focus their efforts there. Whereas in other places, the statistical model is quite accurate, and it’s best to spend effort elsewhere.
So that’s the baseline of how we think about it– again, ever evolving.
We also have an embedded model so we sit with finance and strategy. We work really closely with them and really understand their problems.
It’s very much a partnership-type model rather than a “throw a model over the wall” type model.
Featured in this episode
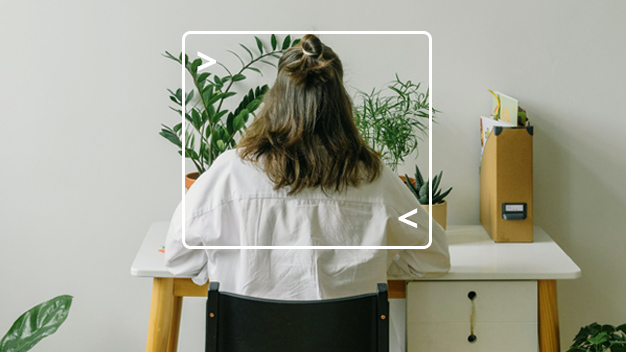
Dara’s team uses forecasting, machine learning, and analytics to solve problems in partnership with the Finance and Operations teams. Before becoming a data scientist, she earned her PhD in Computational Immunology from Stanford and her BA in Biology from Carleton College.