Explicit design at the start of a project
Emily Riederer
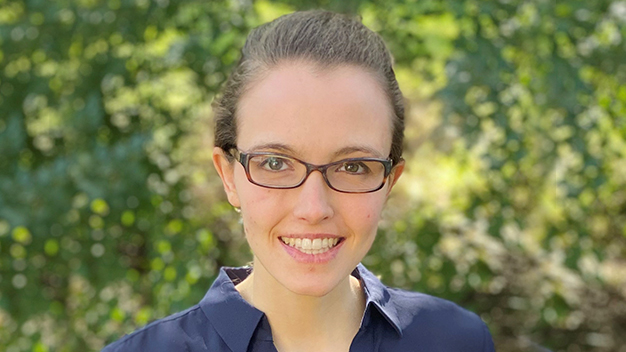
Episode notes
Diving into a question asked at (42:38): What is your thought process for solving a problem that you don’t know how to solve immediately?
One thing that I think is a really undervalued part of that process is thinking about how you will know a good solution when you find one? Also, how would you know if there was a good solution staring you in the face and you already had it?
I think the more unstructured and complicated a problem can be, it can almost be a little deceptive of what’s good– which can have one or two bad outcomes.
You find a good solution, but you don’t realize it’s good so you keep going
You spend a lot of time chasing after an outcome, and only then do you realize, I solved the problem I was trying to solve but it wasn’t the problem I wanted to solve.
Something I’ve really been experimenting with in my own work is having a lot more of an explicit design stage at the beginning of a project and thinking, how can you do a pilot?
If I’m trying to predict some target, can I take those two values of that target and plug them into a downstream problem I actually thought that I was going to solve, and make sure that’s actually what I want to solve?
Almost like frontloading model evaluation with even a fake solution is the first step versus last step.
Then, I’ll check on one other point.
I think the other aspect of that – going back to that level of abstraction – is figuring out how to take the context out of my problem to make it something more Googleable.
So I mean thinking, not being like, “oh, this experiment, the random seeds were wrong, so I don’t have a control population – what do I do?”
Backing that into more of a general question – “how do you sample a synthetic control through observational data?” which is something you can Google and then find a ton of resources about.
I think pushing myself on what I want, and then finding the right framing at which to ask for help.
Featured in this episode
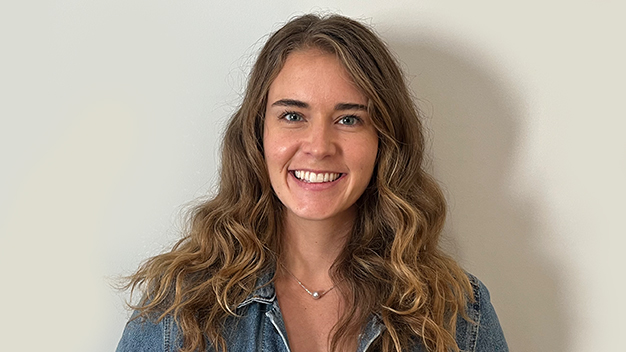
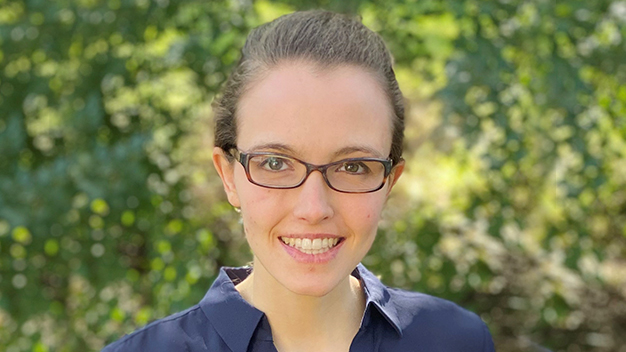
Emily is a senior manager at Capital One where she has built and led a variety focused on all parts of the data science lifecycle -- from strategy and analytics, data infrastructure and reproducible innersource tools, and model development. These diverse roles have made her particularly passionate about how parts of the data lifecycle interact -- for example: how breaking data silos can enable causal inference methods or more efficient data pipelining can unlock creativity in model feature engineering. Outside of work, Emily is a passionate part of the R community.