Building up a data science team in a small org
Everyone
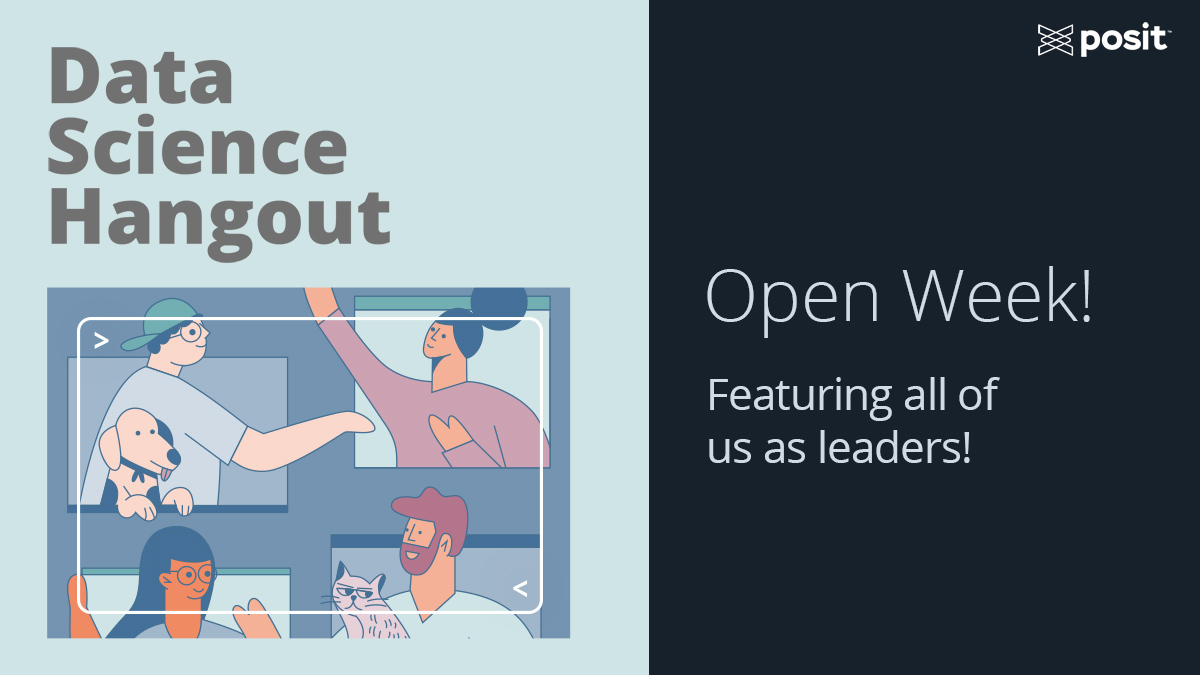
Episode notes
Learning from our first *open week*, we decided we would continue to hold these open weeks once every 2 months or so and we were all the featured leaders for the week, asking questions to each other.
There was a great discussion in the beginning of this week’s hangout on building up a data science team in a smaller organization.
3:53 George shared, “As the first data scientist in HR, I was tasked with starting from scratch with everything. So building out metrics, defining metrics, doing data governance, learning a new HR system, and then from there developing a team. So I think right now it’s just looking at the different tools that are out there, understanding how we might manage a data lake and trying to find the right tools. So far, my approach has been to have discussions with our HR managers – the primary stakeholders and the ones that are going to be leveraging the data – and communicating higher up or to the different managers within the organization. Also thinking about security because with HR data it’s highly secure information that we share. We don’t always want to share demographics, so also thinking about security policies. But just a lot of conversations with people about what their business needs are.
8:11 Eugene shared, “The first question is really what are going to be the highest impact projects and who would I hire as my first employee and then round out based on their weaknesses. But I would aim to try to cover these three bases: a programmer who understands production-level stuff, someone who understands the statistics side (probability distributions for example), and a contextual expert. If you’re really going to build a whole team of four or five, six people, you really need people who are, in my opinion, really good experts at each one of those three things, because it’s very rare to find somebody who’s an expert in all three. You’d be paying out a ton of money if you do find someone who’s an expert in all three.”
9:17, Libby shared that “In interviewing people or interviewing with people she finds that when they are building a team, they’re looking for somebody to hit the ground running. They always use that term: we need somebody to hit the ground running. A lot of times what I feel like they’re saying is we need somebody to do everything and we also need somebody to tell us what to do, because we don’t know what to do. That feels scary because I can’t be everything for everybody, but it also sounds like people are saying that person doesn’t really exist. My take on that is if you have somebody who does everything and knows everything, they are now your single point of failure. If they leave, all of your expertise goes in one person. Instead of needing to replace the one person who was maybe a subject matter expert, you now have nothing.”
10:52, Katherine shared, “I feel like a lot of times, looking for people who can ‘hit the ground running’ is corporate code for we are not going to onboard you or train you at all. When you are building a data science team, you need to say these are the people that I need and the skill sets that they’re going to have and then build your onboarding around what that team is going to look like. One of the things that we’ve been trying to get away from is, ‘oh, well this one person knows everything.’ For six months, all we did was write documentation about our processes and transcribe email chains from three years ago so that when we do bring on new people, we can onboard them not only on the technical aspect of the job, but also on the context of the data. This is especially important because we’re in the medical field, where you are bringing on people who may not have had any medical experience before.”
At 12:06 Coulter shared, “I was in an org where data science was uncharted territory with everything done in Excel. I tried to get buy-in on this from a selfish point of view because I was dealing with all this data and I said, “This shouldn’t take this long. We’re spending a ton of time trying to do all this. Luckily, I had great leadership that said,”You know what, that’s right.” I was able to hire someone and immediately started converting everything to R to automate as many things as possible. The first time that we were able to show someone, hey, you know this thing that used to take you 16 hours, it takes 30 seconds now. And the joy in their faces– I will never forget that. I think that really showed leadership that this is something worth investing in even further. That was 2.5 years ago and now we are a team of 8.”
Featured in this episode
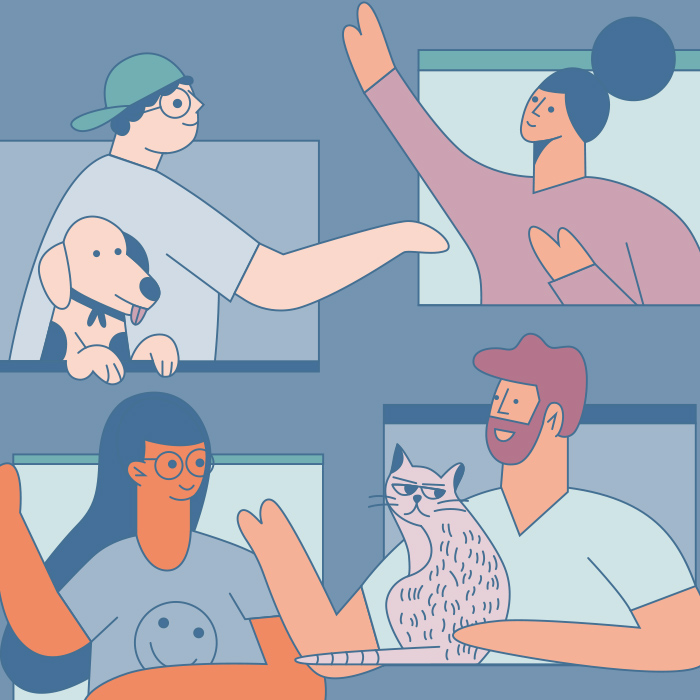
The Data Science Hangout community is made up of people using data (or curious about data) across all different industries, all different roles, and all different levels. This week we hosted everyone on the call as our featured leaders and opened up to a group discussion.