In recent years, numerous highly publicized failures in data science have made evident that biases or issues of fairness in training data can sneak into, and be magnified by, our models, leading to harmful, incorrect predictions being made once the models are deployed into the real world. But what actually constitutes an unfiar or biased model, and how can we diagnose and address these issues within our own work? In this talk, I will present a framework for better understanding how issues of fairness overlap with data science as well as how we can improve our modeling pipelines to make them more interpretable, reproducible, and fair to the groups that they are intended to serve. We will explore this new framework together through an analysis of ProPublica’s COMPAS recidivism dataset using the tidymodels, drake, and iml packages.
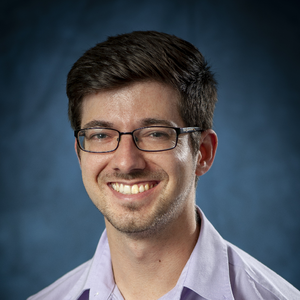
Grant Fleming is a Data Scientist at Elder Research, co-author of the Wiley book _Responsible Data Science_ (2021), and contributor to the O'Reilly book _97 Things About Ethics Everyone in Data Science Should Know_. His professional focus is on machine learning for social science applications, model explainability, and building tools for reproducible data science. Previously, Grant was a research contractor for USAID.