RStudio recently announced a new open-source package called sparklyr that facilitates a connection between R and Spark using a full-fledged dplyr backend with support for the entirety of Spark’s MLlib library. Due to Spark’s ability to interact with distributed data with little latency, it is becoming an attractive tool for interfacing with large datasets in an interactive environment. In addition to handling the storage of data, Spark also incorporates a variety of other tools including stream processing, computing on graphs, and a distributed machine learning framework. Some of these tools are available to R programmers via the sparklyr package.
In this four-part series, we’ll discuss how to leverage Spark’s capabilities in a modern R environment. The sparklyr Series:
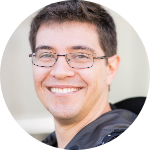
Javier is the author of “Mastering Spark with R”, pins, sparklyr, mlflow and torch. He holds a double degree in Math and Software Engineer and decades of industry experience with a focus on data analysis. Javier is currently working on a project of his own; and previously worked in RStudio, Microsoft Research and SAP.