When tasked with creating the first customer-facing machine learning model at T-Mobile, we were faced with a conundrum. We had been told time and time again to deploy machine learning models in production you had to use Python, but our very best data scientists were fluent in building neural networks in R with Keras and TensorFlow. Determined to avoid double work, we decided to use R in production for our machine learning models. After months of work, wrangling our containers to meet cloud security compliance, and conforming to DevOps standards, we succeeded in creating a containerized API solution using the keras and plumber R packages and Docker. Today R is actively powering tools that our customers directly interact with and we have open sourced our methods. In this talk, we'll walk through how to deploy R models as container-based APIs, the struggles and triumphs we've had using R in production, and how you can design your teams to optimize for this sort of innovation.
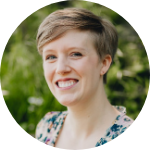
Heather Nolis is a founding member of the AI @ T-Mobile team, focusing the conversion of cutting-edge analyses to real-time, scalable data-driven products. She began her career in neuroscience but once realized how heavily that field relied on software built by other people, she pivoted - deciding to make software herself. You can find her @heatherklus on Twitter, where she speaks about diversity in technology, the ethical implications of data, and cats.